Alessandro Inversini Ecole hôtelière de Lausanne, HES-SO/University of Applied Sciences Western Switzerland, Switzerland. Barbara Neuhofer Salzburg University of Applied Sciences, Austria Thuy Ngoc Nguyen Free University of Bozen-Bolzano, Italy. Sangwon Park Hong Kong Polytechnic University, Hong Kong Katja Pasanen University of Eastern Finland, Finland.
Mattia Rainoldi Salzburg University of Applied Sciences, Austria Gang Ren Pusan National University, South Korea. Marko Tkalcic Free University of Bozen-Bolzano, Italy Pasi Tuominen Haaga-Helia High School.
Clustering Users’ POIs Visit Trajectories for Next-POI Recommendation
1 Introduction
In order to predict relevant POIs, the first approach exploits a combination of contextual factors with explicit user feedback, e.g. POI ratings. The second extracts visit patterns in the POI visit trajectories of the observed user to predict the next POI the user will visit. In addition, both approaches require a lot of observations (e.g. POI scores or trajectories) to generate recommendations.
In the approach proposed here, first users are grouped based on similarities in their observed POI visit trajectories. This paper extends [6] by using a much larger database of user POI visit trajectories and using a new implementation of the behavioral learning algorithm that uses GPU hardware.
2 Related Work
Finally, next POI recommendations for users in a cluster are generated based on the behavioral model learned on that cluster. Clusters of users' POIs Visit trajectories for next-POI recommendation 5 that relevant POIs can then be retrieved from a database using the predicted next-POI category according to different strategies (e.g. popularity or price ). Here, clustering solves the new user problem, but it does not support learning the user behavior model as proposed in this paper.
In [8], the authors aim to find an optimal route recommendation based on distance and travel time. So, unlike the approach proposed here, no recommendation technique for new POIs is proposed in [8] and clustering is applied to POIs rather than to trajectories.
3 Trajectory Clustering and Recommendation
Sequential Decision Making Model
POIs Visit Trajectories Clustering
In fact, the POI circles in the cluster are represented on the right smaller due to a more uniform distribution of the visits among all the POIs in the cluster (ie not only the top-15 shown in the figure). The importance of the different streets in the clustered lanes is determined by identifying the most representative lanes in the clusters. At the bottom of Fig.1, the ranked lists of the most popular POIs in the two clusters are shown.
This figure shows the probability of different features (POI category, historical period and associated person characteristics) in the five clusters considered. For example, POI categories such as churches and buildings mainly characterize visits to clusters A and D, while to a lesser extent trajectories in other clusters (eg, cluster E).
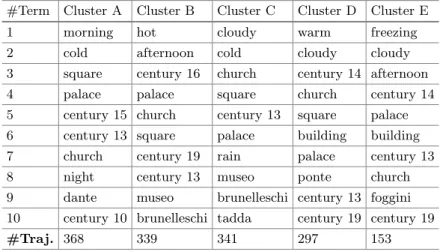
Next-POI Visit Recommendation
For example, it can be seen that groups C and E mainly cluster visits during cloudy days (left). For example, cluster A represents visits on cold days, while cluster C represents visits on warm days. Interestingly, focusing on the day part (right), there are groups representing visits made at different times.
The user-specific preference model is built by observing the frequencies of the descriptive features (eg, POI category) that appear only in the POIs of the user's observed trajectories. The overall scores for alternative next POI visits are generated by a linear combination of the user-specific POI scores and the group-specific scores proportional to the Q value of the visits (general model).
4 Recommender System Evaluation
The optimal action, as explained in Section 3.1, is the one with the highest Q value in the user's current state. When multiple observations of visiting actions are available for a user, recommendations can also be generated by combining the group generalized user behavior (as used in the previous strategy) with the results of visiting the next POI calculated based on the user's preference model.
5 Experimental Results
6 Conclusion
Characterisation of Traveller Types Using Check-In Data from Location-Based
Social Networks
Tourist Recommender Systems
Recommender systems for tourism are a well-established tool due to the considerable complexity of planning an independent trip and the great economic importance of the travel and tourism industry. Since these items are often not as well defined as hotels or restaurants, collaborative filtering methods are not commonly used. Characterizing traveler types using check-in data 17 To facilitate the content-based paradigm, user needs are mapped onto item attributes.
This categorization problem is not trivial as it requires reliable information about the user and the candidates.
Tourist Roles
Human Mobility Analysis
3 From Check-Ins to Human Mobility Patterns
Choosing Features for Clustering
Trip duration: the number of days between the first and the last check-in of the trip. Check-in time: the average time of day of the check-in time, indicating the time of activity. As shown in Fig.1, there is a strong positive correlation between the radius of gyration and the check-in distance, with a coefficient of 0.74.
Therefore, the latter is excluded, since the rotation radius is less dependent on the user's input behavior. Day length and entry time are barely correlated (≤ ±0.11) with the other traits.
4 Clustering Results
In addition to the day length, the check-in time and the mean displacement all follow an exponential distribution with a long tail, which limits the interpretability of the mean and median values. Before applying the clustering algorithm, a correlation analysis of the remaining candidate features is performed. As shown in Fig.2a, the average silhouette of the hierarchical clustering is in the area of slightly above 0.5 for two to four clusters and peaks below 0.4 at five clusters or more.
A quick look at the silhouette plots of the four clusters in Fig.2b shows that they are mostly in the positive zone, with few. group trips that fall into the negative zone. The overall mean displacement was large, but the radius of gyration was relatively small, as the entire trip took place in the northeast of the United States of America (see Fig.3b). a) Travel of a Vacationer (b) Travel of an Explorer.

5 Conclusions and Future Work
The user can check the crowd information of the restricted places (pci Pc); the system provides daily crowd charts. Using crowd information from tourist spots in an interactive 31. draws out the perception of the tourist to the crowd pci. The end of the visiting time frame differs from the closing time of the location.
Let's say the end of the visit period + expected time of stay/2 are the place's virtual business hours. The proposed CT-Planner offered alternative plans in 70% of the cases based on the user's visiting time.

Decision Making Based on Bimodal Rating Summary Statistics - An
Eye-Tracking Study of Hotels
A rank-based conjoint experiment, complete with eye-tracking, was conducted to measure participants' focus of attention and understand how they evaluate emerging differences in their decision-making process. The literature on recommender systems recognizes that decision-making strategies vary from person to person. In the field of recommender systems, relatively few studies consider the maximization of the decision-making process.
Bart Knijnenburg and his colleagues [8] controlled interfaces in a between-subjects experiment to trigger different decision-making strategies. Conjoint analysis is a widely appreciated methodological tool of marketing and consumer research, which is particularly useful for studying user preferences and trade-offs in the decision-making process [14].
3 Methodology
Study Procedure
Participants (who gave informed consent for their data to be used for research purposes) were asked to complete the abbreviated Maximization Scale described above. Given the above, which of the hotels below would you prefer if you were to consider only the ratings for the displayed accommodation. 2 Note that the original, outdated phrase Rent a video[.]in the scale of [31] has been replaced in the current research with Book a hotel[.].
In each task, respondents had to rank 6 of 18 items from the full factorial combinations of the attribute levels. The order of the six items displayed (i.e., the profiles) on a single screen was randomized for each respondent.
4 Results
Decision Making Based on Bimodal Rating Summary Statistics 47 After this introduction, the participant went through 3 consecutive ranking tasks. Presented profiles were item-agnostic, so users could base their selection solely on the three study variables (i.e., number of ratings, mean, and bimodality). Figure 5 shows the geometric mean of the fixation times on the items arranged according to the ranking positions they received from the respective participant, and grouped according to the median distribution on Decision Difficulty.
5. Geometric mean of time spent on the item (95% confidence level), mean split on the decision difficulty subscale. Accordingly, Fig.6 shows the mean number of revisits—that is, how often the participant's gaze switched between an AOI of one item and an AOI of another item, forward and backward—grouped by mean separation and ranked by rank currently assigned by the participant.

5 Discussion and Conclusions
Most research on the sharing economy has analyzed the consumer's point of view. Finally, and probably most importantly, the analysis focused on the global problem of the sharing economy. An analysis of the spatial distribution of Airbnb ads in Barcelona by Gutiérrez et al.
Zervas G, Proserpio D, Byers J (2016) The rise of the sharing economy: assessing the impact of Airbnb on the hotel industry. I had the feeling that I was in the center of the action and not just watching. Model fit of the structural model was evaluated based on the comparative fit index (CFI), non-normed fit index (NNFI), standardized root mean square residual (SRMR), and root mean square error of approximation (RMSEA).
Figure 2 serves as a summary of the most frequent keywords of each section (from Table 5) by integrating the words that convey good and bad feelings and by integrating the results into two result categories (score+ and score−). However, it is difficult to confirm that related studies in the literature have met all criteria. Figure 2 shows a visualization of three dimensions with destinations and themes of experiences.
